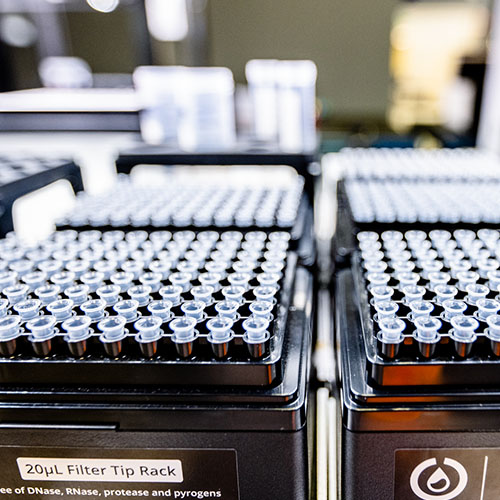
The RPL has developed a groundbreaking method that leverages robotics to automate the preparation, characterization, and disposal of complex liquid samples for synchrotron coherent X-ray scattering experiments. Using a pendant drop technique shielded from air turbulence, the automated experiments achieved results consistent with traditional containers, making this approach suitable for high-precision studies. The robotic system, featuring an electronic pipette mounted on a robotic arm, enables precise sample handling and high-throughput exchange. By integrating a single Python script with beamline and robot control libraries, this enables seamless automation, paving the way for AI-driven, fully autonomous material design at large-scale scientific facilities. This approach enhances experimental efficiency and consistency, revolutionizing the study of complex fluids. Read the related paper.